Le cycle des séminaires scientifiques ANITI continue.
Rendez-vous le 21 janvier de 15h à 16h en ligne avec George Karniadakis pour un séminaire autour de l’approximation de fonctions, des fonctionnelles et des opérateurs avec des réseaux de neurones pour diverses applications.
A propos de George Karniadakis
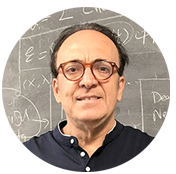
George Karniadakis est professeur de mathématiques appliquées à l’Université de Brown. C’est un chercheur gréco-américain connu pour ses travaux à large spectre sur la modélisation stochastique de grande dimension et les simulations multi-échelles de systèmes physiques et biologiques pour la quantification de l’incertitude et la théorie de Sturm-Liouville pour les équations aux dérivées partielles et le calcul fractionnaire.
Résumé (en anglais) :
We will review physics-informed neural network and summarize available extensions for applications in computational mechanics and beyond. We will also introduce new NNs that learn functionals and nonlinear operators from functions and corresponding responses for system identification. The universal approximation theorem of operators is suggestive of the potential of NNs in learning from scattered data any continuous operator or complex system. We first generalize the theorem to deep neural networks, and subsequently we apply it to design a new composite NN with small generalization error, the deep operator network (DeepONet), consisting of a NN for encoding the discrete input function space (branch net) and another NN for encoding the domain of the output functions (trunk net). We demonstrate that DeepONet can learn various explicit operators, e.g., integrals, Laplace transforms and fractional Laplacians, as well as implicit operators that represent deterministic and stochastic differential equations. More generally, DeepOnet can learn multiscale operators spanning across many scales and trained by diverse sources of data simultaneously.