Chair objectives
The purpose of the chair is to design novel and improved neural network/deep learning architectures by drawing inductive constraints from various domains: for example, semantic constraints from NLP (Natural Language Processing) models can improve computer vision via semantic grounding; cognitive architectures emulating the human mind can help towards flexible AI systems; biological inspiration from neuroscience can ensure that our AI models are more similar to human brains, with human-compatible representations or values.
Program Collaborative AI
Themes: Learning with little or complex data, language, neuroscience & AI, robotics & AI
Chair holder:
Rufin Van Rullen, DR CNRS, CerCo
Co-chairs:
- Leila Reddy (CR CNRS,
- Francis Filbet (Prof. UT3),
- Gregory Faye (CR CNRS)
- Chloé Braud (CNRS IRIT)
Chair holder: Rufin VanRullen (CNRS, CerCo)
Co-chairs
- Chloé Braud (CNRS, IRIT)
- Grégory Faye (CNRS, IMT),
- Francis Filbet (UT3, IMT),
- Leila Reddy (CNRS, CerCo)
PhD students
- Romain Bielawski, 09/2019-2022
- Benjamin Devillers, 09/2019-2022
- Mathieu Chalvidal, 01/2020-2023 (50% with T. Serre’s chair)
- Colin Decourt, 09/2020-2023 (50% with N. Dobigeon’s chair)
- Europe: ANR COCOBOTS France-Germany 2021-2024 in negotiation phase
- France: ANR AI-REPS 2018-2022 (PI: L. Reddy, co-PI N. Asher)
- Other: ANR OSCI-DEEP 2019-2022 France-USA with T. Serre
- Notre chaire porte le thème “Language” d’ANITI (de nombreuses rencontres organisées)
- Organisation d’une classe Intro2AI en 2 sessions en 2021 :
en savoir +
- Devillers, B., Choksi, B., Bielawski, R., & VanRullen, R. (2021). Does language help generalization in vision models?. arXiv:2104.08313 (presented at ViGIL workshop at NAACL 2021)
- VanRullen, R., & Kanai, R. (2021). Deep learning and the Global Workspace Theory. Trends in Neurosciences.
- VanRullen, R., & Alamia, A. (2021). GAttANet: Global attention agreement for convolutional neural networks. arXiv:2104.05575 (to appear in ICANN 2021).
- VanRullen, R., & Reddy, L. (2019). Reconstructing faces from fMRI patterns using deep generative neural networks. Communications biology, 2(1), 1-10.
- Mozafari, M., Reddy, L., & VanRullen, R. (2020). Reconstructing natural scenes from fMRI patterns using BigBiGAN. In 2020 International Joint Conference on Neural Networks (IJCNN) (pp. 1-8). IEEE.
- Reddy, L., Cichy, R. M., & VanRullen, R. (2021). Representational Content of Oscillatory Brain Activity during Object Recognition: Contrasting Cortical and Deep Neural Network Hierarchies. eNeuro, 8(3).
- Choksi, B., Mozafari, M., O’May, C. B., Ador, B., Alamia, A., & VanRullen, R. (2021). Predify: Augmenting deep neural networks with brain-inspired predictive coding dynamics. arXiv preprint arXiv:2106.02749.
- Alamia, A., Mozafari, M., Choksi, B., & VanRullen, R. (2021). On the role of feedback in visual processing: a predictive coding perspective. arXiv preprint arXiv:2106.04225
- Z Pang, CB O’May, B Choksi & VanRullen, R. (2021). Predictive coding feedback results in perceived illusory contours in a recurrent neural network. arXiv preprint arXiv:2102.01955
Know more
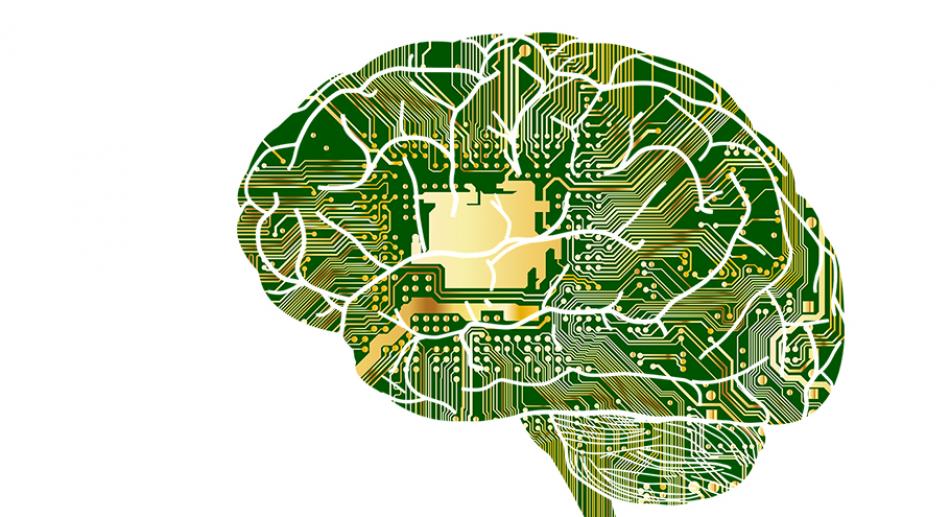
Biomimicry: the brain's promises for AI
To be inspired by living things to imagine solutions and inventions produced by nature: this is the object of biomimicry, this process of innovation that is required in most sectors of human activity. In artificial intelligence (AI), cognitive neurosciences are a privileged source of inspiration. Demonstration with CNRS researcher Rufin VanRullen and deputy director of the European Center of Excellence in Biomimicry, Laura Magro.
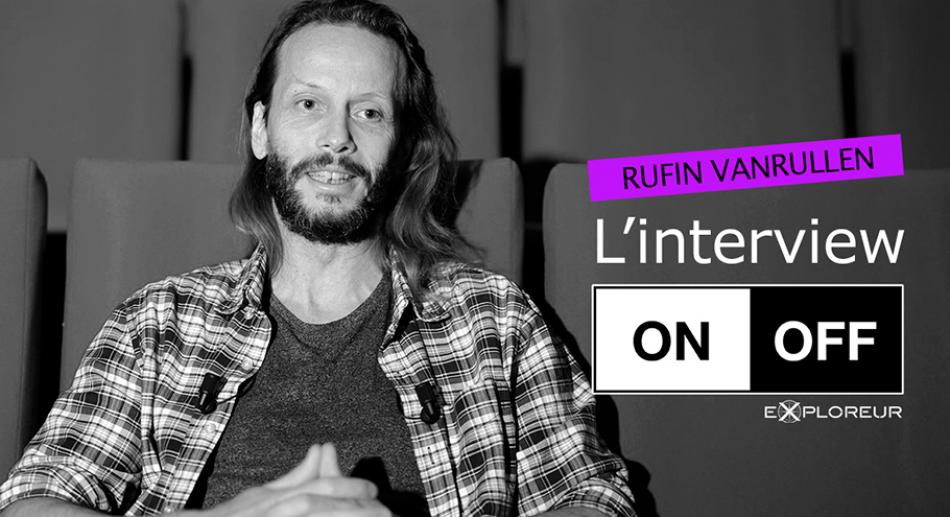
Rufin VanRullen, the brain-inspired researcher
Expert in neuroscience, Rufin VanRullen is inspired by the functioning of the brain to create increasingly efficient artificial neural networks…. And dreams of intelligent machines.