Chair objectives
The goal of the Chair is to build new methods in machine learning to obtain fair and robust
algorithms. The presence of bias and discrimination is well acknowledged in machine learning.
Instead of providing decisions which appear as sharp and accurate, algorithms may perpetuate or even exacerbate biases in the training data.
The goal of this project is to develop new types of machine learning algorithms, which, while being able to provide efficient forecasts or predictions, do not reflect any bias in their output and thus achieve what is nowadays called fairness in algorithmic
decisions.
The number of research papers in this field has grown exponentially over the last few
years and recent contributions to the field have proposed various mathematical definitions and novel algorithms for addressing fairness in a wide range of learning problems. Yet few works have managed to derive algorithms which are supported by strong theoretical guarantees and the quality assessment, which is an important requirement to be able to certify fair behaviour of an algorithm.
Programs: Acceptable, certifiable & collaborative AI
Themes: Explainability, Fair Learning
Chair holder:
Jean-Michel Loubès, PR UT3, IMT
Senior collaborating researchers
Matthieu Serrurier (UT3, IRIT),
Béatrice Laurent (INSA Toulouse, IMT)
Chair holder
Jean-Michel Loubes, (UT3, Institut de Mathématiques de Toulouse-IMT)
Senior collaborating researchers
Mathieu Serrurier (UT3, IRIT),
Béatrice Laurent (INSA Toulouse, IMT)
PhD students:
Alberto Gonzalez (with E. Del Barrio, funded by ANITI)
C. Benesse (with F. Gamboa, funded by ENS)
L. De Lara (with L. Risser and N. Asher, funded by Ecole Polytechnique) 4/ W. Todo (with B. Laurent,funded by Liebher in CIFRE)
Visiting researchers
Del Barrio Eustasio, University of Valladolid, February 2020
- Europe: COALA
- France: Confiance.ai, DEEL project
- E Del Barrio, P Gordaliza, JM Loubes, A central limit theorem for Lp transportation cost on the real line with application to fairness assessment in machine learning, Information and Inference: A Journal of the IMA 8 (4), 817-849. 2019
- P Gordaliza, E Del Barrio, G Fabrice, JM Loubes, Obtaining fairness using optimal transport theory, International Conference on Machine Learning, 2357-2365, 2019.
- Can Everyday AI be Ethical? Machine Learning Algorithm Fairness P Besse, C Castets-Renard, A Garivier, JM Loubes Machine Learning Algorithm Fairness (May 20, 2018). Statistiques et Société
- M. Serrurier, F. Mamalet, A. González-Sanz, T. Boissin, JM Loubes, Achieving robustness in classification using optimal transport with hinge regularization Proceedings of the IEEE/CVF Conference on Computer Vision and Pattern (2021)
- E Del Barrio, JM Loubes, Central limit theorems for empirical transportation cost in general dimension, The Annals of Probability 47 (2), 926-951 (2019)
- E del Barrio, A González-Sanz, JM Loubes, A Central Limit Theorem for Semidiscrete Wasserstein Distances, arXiv preprint arXiv:2105.11721 (2021)
- E del Barrio, A González-Sanz, JM Loubes, Central Limit Theorems for General Transportation Costs, arXiv preprint arXiv:2102.06379 (2021)
- J Lam-Weil, B Laurent, JM Loubes, Minimax optimal goodness-of-fit testing for densities under a local differential privacy constraint, – Bernoulli, 2021
- L de Lara, A González-Sanz, N Asher, JM Loubes, Counterfactual Models: The Mass Transportation Viewpoint, 2021
- F Bachoc, F Gamboa, M Halford, JM Loubes, L Risser, Entropic Variable Projection for Explainability and Intepretability, arXiv preprint arXiv:1810.07924, 2020.
- C Bénesse, F Gamboa, JM Loubes, T Boissin, Fairness seen as Global Sensitivity Analysis, arXiv preprint arXiv:2103.04613, 2021
Know more
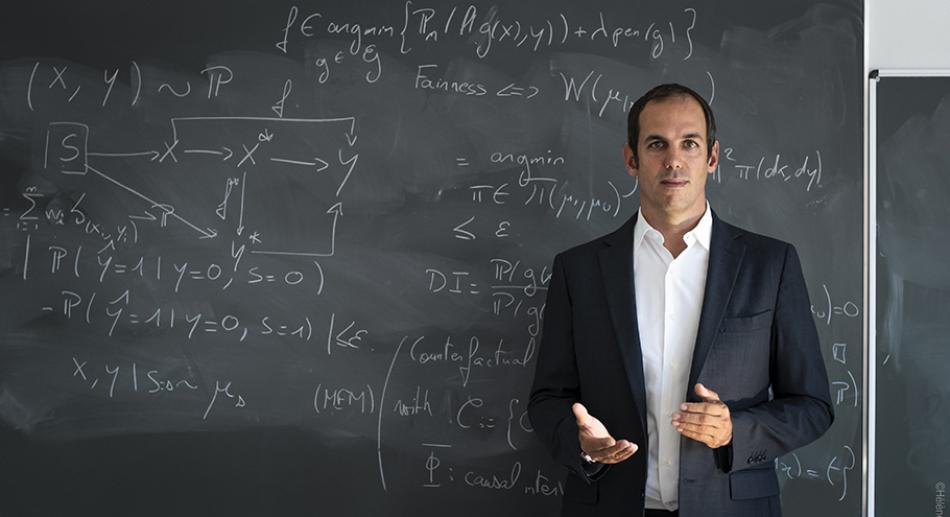
Jean Michel Loubès, the mathematician who teaches machines
An Exploreur article
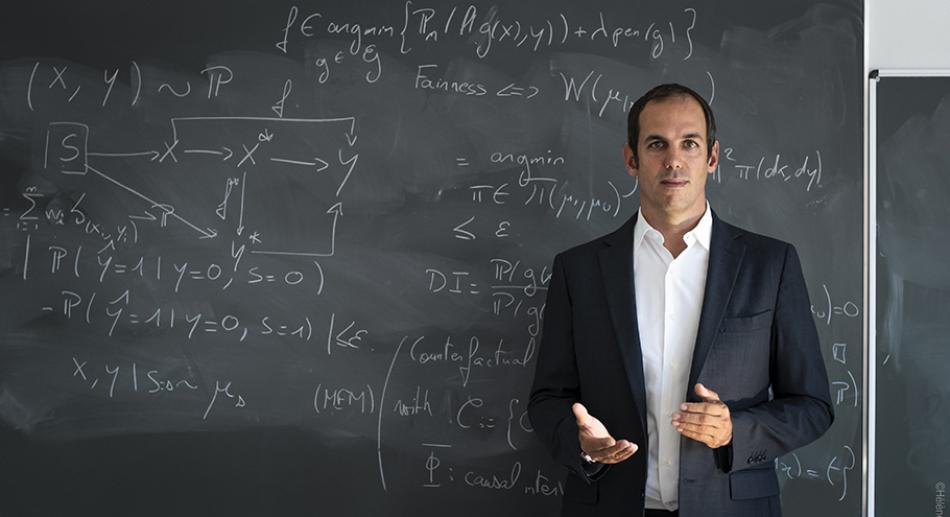
Jean-Michel Loubes, les algorithmes pourraient rendre la société plus équitable.