Chair objectives
L’objectif de la chaire est de développer et appliquer des technologies hybrides d’IA dans le contexte de la conception, et plus précisément la conception moléculaire (protéique) avec une cible qui couvre l’ensemble des spectres qui vont du développement algorithmique à l’application à de vraies molécules qui seront testées expérimentalement.
Designing requires to build original objects satisfying constraints (physical and
design constraints) in an existing family of designs from which knowledge can be extracted by Machine and Deep Learning.
Nous souhaitons combiner le raisonnement automatisé, en utilisant le Solveur de problèmes de satisfaction de contraintes (WCSP) (toulbar2) développé à Toulouse, afin de résoudre les problèmes de conception de base (combinaison de contraintes et de critères), avec du Machine Learning qui capture « orientations de conception » (critères) à partir des conceptions/solutions existantes ainsi que du Deep Learning, utilisé pour ses capacités perceptives et non linéaires.
Programs: Acceptable, certifiable & collaborative AI
Thèmes : Learning with little or complex data, AI and physical models, automated reasoning and decision making, explainability
Chair holder:
Thomas Schiex, DR Inra, MIAT
Co-chairs:
- Sophie Barbe, INSA Toulouse,
- David Simoncini, Irit
- Simon de Givry (INRAE, MIAT
- George Katsirelos (INRAE, MIAT)
Chair holder : Thomas Schiex, INRAE
Co-chairs
- Simon de Givry (INRAE, MIAT)
- George Katsirelos (INRAE, MIAT)
- Sophie Barbe (INRAE, Toulouse Biotechnology Institute), David Simoncini (UT1, IRIT)
PhD students
- Valentin Durante (2020-), Pierre Montalbano (2020-) via ANITI.
- Mariane Defresne (SEVAB – BIOEco & ANITI labelled), Manon Ruffini (ENS Rennes), Jelena Vucinic (INRAE/R.gion). Y. Bouchiba (SEVAB)
Thomas Schiex : AAAI Fellow 2020
- Improved Acyclicity Reasoning for Bayesian Network Structure Learning with Constraint Programming, F. Trösser, S. de Givry, G.Katsirelos. IJCAI 2021
- Pushing data into CP models using Graphical Model Learning and Solving. C. Brouard, S. de Givry, T. Schiex. CP’2020
- Guaranted Diversity and Optimality in Cost Function Network Based Computational Protein Design Methods. M. Ruffini, J. Vucinic, S. de Givry, G. Katsirelos, S. Barbe, T. Schiex. Algorithms, 2021.
- Positive Multi-State Protein Design. J. Vučinić, D. Simoncini, M.Ruffini, S . Barbe, T. Schiex. Bioinformatics (2020).
- Computational design of symmetrical 8-bladed bêta-propeller proteins. Noguchi, H., Addy, C.,Simoncini, D., Wouters, S., Mylemans, B., Van Meervelt, L., Schiex, T., Zhang, K.Y.J., Tame, J.R.H. & Voet, A.R.D. (2019). IUCrJ 6.
- A comparative study to decipher the structural and dynamics determinants underlying the activity and thermal stability of GH-11 xylanases. J. Vucinic, G. Novikov, C. Montanier, C. Dumon, T.Schiex and S. Barbe. Molecular Biophysics, Computational Studies of Biomolecules, II. June 2021.
- Seven amino acid types suffice to reconstruct the core fold of RNA polymerase. S. Yagi, A.K Padhi, J. Vucinic, S. Barbe, T. Schiex, R. Nakagawa, D. Simoncini, K.Zhang, S. Tagami. bioRxiv, 2021.
- Computational Design of miniprotein binders. Y. Bouchiba, M.Ruffini, T. Schiex, S. Barbe. To appear as a chapter in a book on Peptide Design, Methods in Enzymology Series, Springer, 2021
- Molecular flexibility in computational protein design: an algorithmic perspective. Y. Bouchiba, J.Cortés, T. Schiex, S. Barbe Protein Engineering Design and Selection, May 2021.
- Iterated Local Search with Partition Crossover for Computational Protein Design. F Beuvin, S de Givry, T Schiex, S Verel, D Simoncini. PROTEINS: Structure, Function, and Bioinformatics, May 2021.
- Protein design by provable algorithms. Hallen, M. A., & Donald, B. R. (2019). Communications of the ACM, 62(10), 76-84.
- Designing peptides on a quantum computer. Mulligan, Vikram Khipple, et al. bioRxiv (2020): 752485.
Know more
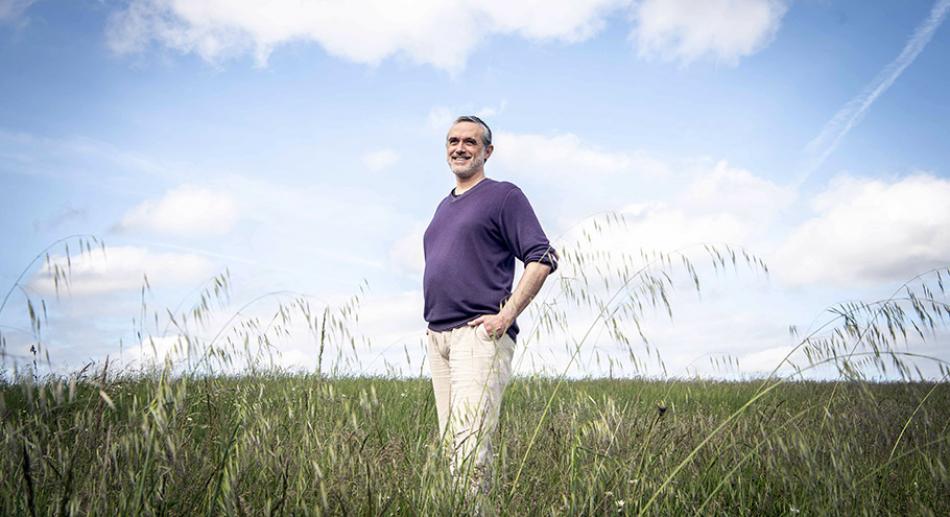
Biology and AI: the virtuous duo of Thomas Schiex
AI must serve humanity. Applied to biology, algorithms offer great opportunities for applications. Meeting with Thomas Schiex, the bioinformatician who links theory to practice.
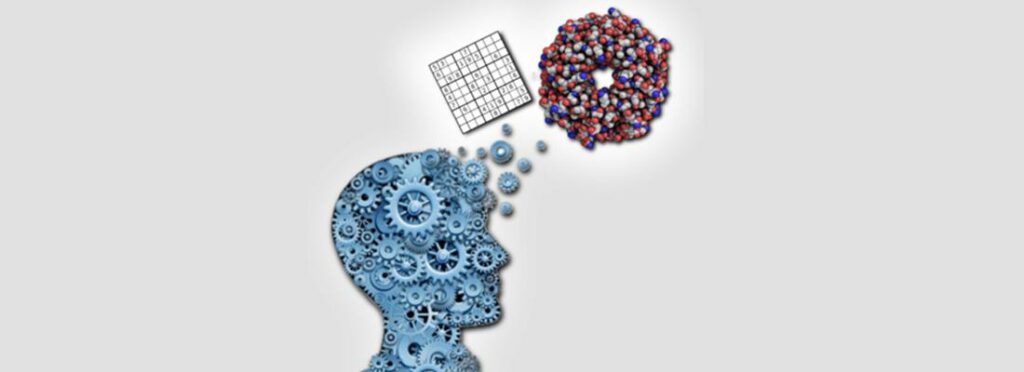
Café du quai: "How artificial intelligence solves (the most serious) puzzles"
Thomas Schiex, INRAE Occitanie-Toulouse researcher presented his research work at the Quai des savoirs as part of the "Explorer Meetings" offered by the Interdisciplinary Institute of Artificial Intelligence of Toulouse (ANITI).
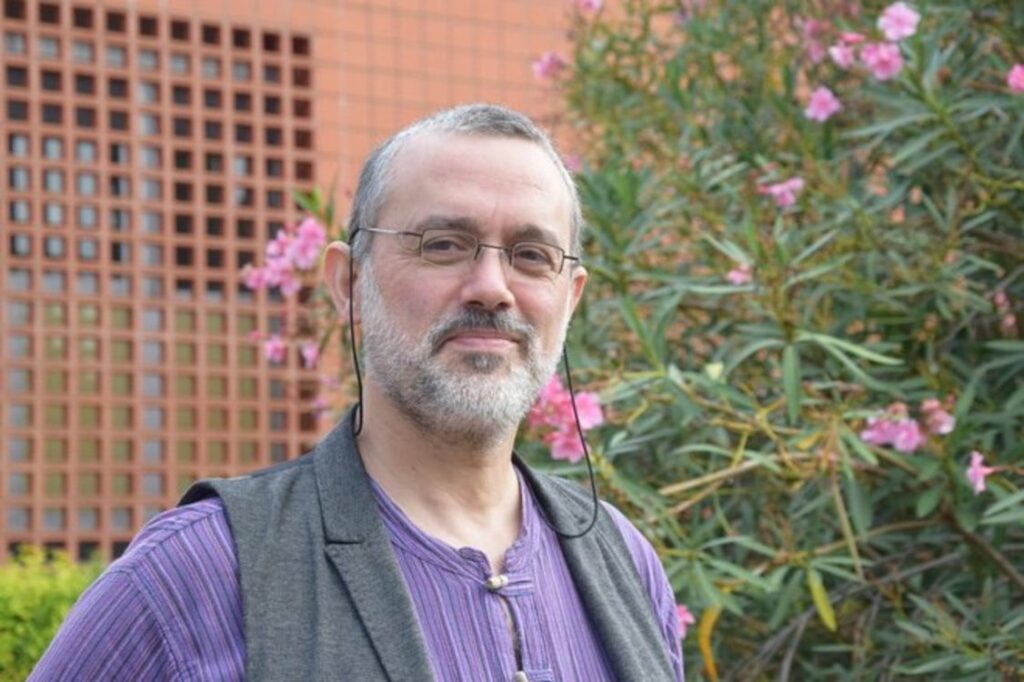
Thomas Schiex receives the EurAI Fellows, for his exceptional work in the service of Artificial Intelligence