Objectives of the chair
The aim of this project is to use symbolic AI approaches, namely (modal) logic and
argumentation theory, for explaining and improving predictions of data-driven models.
We are particularly interested in different types of classifiers (binary, multi-class, and multi-label), and in both black-box models
(whose internal reasoning is left unspecified) and white-box models including Naive Bayes, Decision Trees, and
Random Forest.
The chair has four main research threads:
- Theoretical foundations of explainability
- Explainability of black-box models
- Explainability of white-box models
- Natural Language Processing
Programs: Acceptable, certifiable & collaborative AI
Themes: Explicability, Fair Learning
Chair holder:
Leïla Amgoud, DR CNRS, IRI
Senior collaborating researchers
Emiliano LORINI (CNRS, IRIT),
Philippe MULLER (UT3, IRIT)
Chair holder
Leila Amgoud (DR CNRS, IRIT)
Senior collaborating researchers
- Emiliano LORINI (CNRS, IRIT)
-
Philippe MULLER (UT3, IRIT)
PhD students:
- Vivien Beuselinck, Nov. 2020 – Dec. 2023
- Xinghan Liu, Nov. 2020 – Dec. 2023
- Henri Trenquier, June. 2020 – July 2023
Post-docs
-
Zoe Purcell 2020
-
Bence Bago 2020
2 ANR projects (CoPains, Slant):
- Cognitive Planning in Persuasive Multimodal Communication (CoPains), 2019 – 2021.
- Spin and Bias in Language Analyzed in News and Text (Slant), 2020 – 2022.
CNRS@CREATE Program DesCARTES (France – Singapore).
DesCartes: AI-based Decision making in Critical Urban Systems.
- L. Amgoud. Associate editor of Artificial Intelligence Journal (AIJ)
- L. Amgoud. Member of AIJ Awards Committee (since January 2021)
- Philippe Muller: scientific mediation: participation to the workshop “Groupe Artistique d’Exploration Scientifique”, Quai des savoirs, Toulouse, June 2021
- Leila Amgoud, Dragan Doder, Srdjan Vesic. Evaluation of argument strength in weighted graphs: Foundations et semantics. In Artificial Intelligence Journal, 2021.
- Leila Amgoud, Victor David. A general setting for gradual semantics dealing with similarity. In AAAI-2021, p.6185-6192.
- Leila Amgoud, Vivien Beuselinck. Equivalence of semantics in argumentation. In KR-2021.
- Leila Amgoud. Non-Monotonic explanation functions. In ECSQARU-2021.
- Leila Amgoud. Explaining black-box classification models with arguments. KR-2020 (poster)
- Leila Amgoud. Evaluation of analogical arguments by Choquet integral. In ECAI-2020, p. 593-600.
- Leila Amgoud. Axiomatic Foundations of Explainability. June 2021 (submitted paper).
- Leila Amgoud. A Replication Study of Semantics in Argumentation. IJCAI-2019, p. 6260-6266.
- Jorge Luis Fernandez Davila, Dominique Longin, Emiliano Lorini, Frédéric Maris. A Simple Framework for Cognitive Planning. In AAAI-21, p. 6331-6339.
- Emiliano Lorini, François Schwarzentruber. A Computationally Grounded Logic of Graded Belief. In JELIA- 2021, p. 245-261.
- Emiliano Lorini. Rethinking epistemic logic with belief bases. Artificial Intelligence Journal, 2020.
- Liu, X., Lorini, E. (2021). A logic for binary classifiers et their explanation. CoRR abs/2105.14452.
- Tom Bourgeade, Philippe Muller et Tim Van de Cruys : Plongements Interprétables pour la Détection de is Cachés, TALN 2021.
Know more
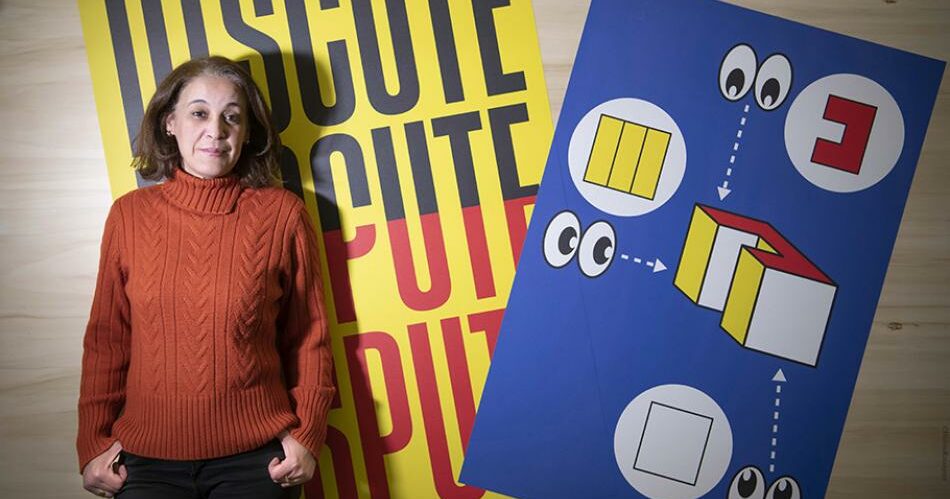