Objectives of the chair
The chair focuses on the training part of neural networks, “intelligence acquisition”, and on the mathematics of the learning phase.
We seek for adequate mathematical tools to assess efficiency in training, learning and forms of robustness.
Our angles for achieving such goals range from the consideration of structural properties of neural networks, mathematical analysis, to algorithm design.
The three lines along which we address these issues are:
- the geometry of neural networks
- the tools for nonsmooth optimization (in particular, the mathematics of algorithmic differentiation)
- the geometry/study of algorithms for the training phase
Our scientific approach mixes: mathematics (optimization, geometry, analysis), computer science, and applications to signal processing.
Program Certifiable AI
Themes: AI and physical models, Optimization and game theory for AI
Chair holder:
Jérôme Bolte, Université Toulouse Capitole
Co-chairs
F. de Gournay (Insa)
F. Malgouyres (UT3),
E. Pauwels (UT3),
P. Weiss (CNRS),
Sites
Chair holder : Jérôme Bolte, TSE, Université Toulouse 1 Capitole
Co-chairs:
- François Malgouyres (UT3, IMT)
- Edouard Pauwels (UT3, IRIT)
Senior collaborating researchers
- Frédéric de Gournay (INSA Toulouse, IMT),
- Pierre Weiss (CNRS, IMT)
PhD students
- Radu Dragomir (JB)
- Joachim Bona-Pellisier (FM)
- El Mehdi Achour (FM)
- Tam Le Ngoc (JB, EP)
Post-docs
- Lilian Glaudin (JB and EP)
- Rodolfo Rios-Zertuche (JB and EP)
- Cyrille Combettes (JB and EP)
- Antonio Siveti-Falls (JB and EP)
Visiting researchers
- Shoham Sabach (Haifa)
- Marc Teboulle (Tel Aviv U.)
- Swann Marx (E. Pauwels)
- Bronze medal in Computer Science for E. Pauwels
- Agenium space: P. Weiss wins "SpotGeo" Challenge Satellite Detection with Deep Learning augmentation of low cost telescope
- J. Bolte appointed AE in Foundations of Computational Mathematics
- J. Bolte appointed AE in SIAM Optimization
- One World Optimization Seminar (2020)
- Poema European network learning week (2020)
- Tutorial at Games, Approachability and Learning Workshop (2021)
- Mathematical colloquium of the University of Hong Kong (2021)
- E. Achour, F. Malgouyres, S. Gerchinovitz, Global minimizerss, strict and non-strict saddle points, and implicit regularization for deep linear neural networks, submitted to FOCM
- J. Bolte, E. Pauwels. Conservative set valued fields, automatic differentiation, stochastic gradient methods and deep learning. Mathematical Programming (2020): 1-33.
- Bolte, J., & Pauwels, E. (2020). A mathematical model for automatic differentiation in machine learning, NeuRIPS 2020
Know more
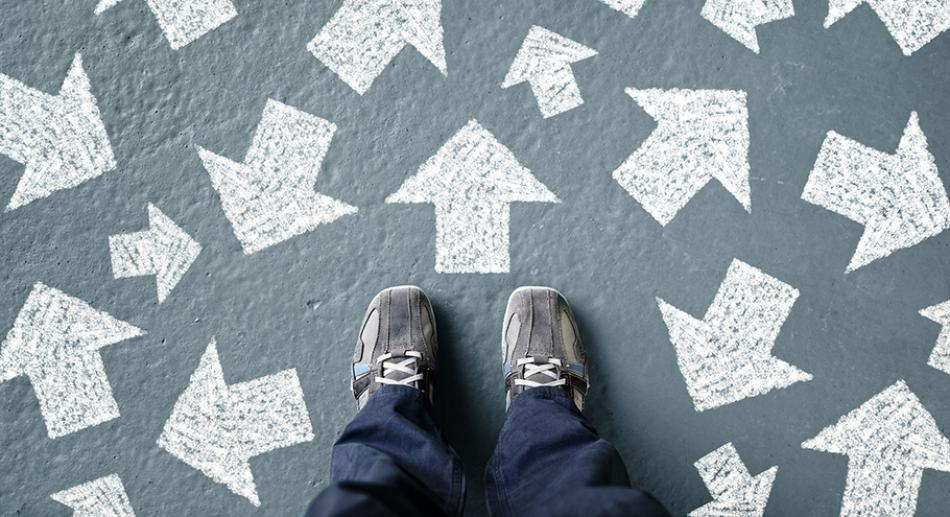
In artificial intelligence, there is no point in running, you have to take the right path
In the mathematics family, optimization is a discipline in its own right that is essential for research in artificial intelligence. Explanations with researcher Jérôme Bolte, holder of the "Large-scale optimization for AI" chair.