Chair objectives
Exploitation and analysis of heterogeneous data are usually conducted thanks to specific methods, generally dedicated to each kind of data, which account for the measurement process and the very nature of the data itself.
Within advanced scenarii of concomitant availability of several yet distinct measurements, fully analyzing these extended datasets requires a unifying framework overcoming a crude and marginal description of a single measurement.
The main objective of this research program is to develop learning algorithms able to extract meaningful information from multi-source, multi-scale and multi-temporal data. Particular applicative contexts deal with remote sensing for Earth observation and automotive systems.
Programs Acceptable & certifiable AI
Themes: Learning with little or complex data, AI and physical models
Chair holder: Nicolas Dobigeon, Toulouse INP
Senior collaborating researchers
- Mathieu Fauvel, INRAE
- Cédric Févotte, CNRS
- Jodi Inglada, Cnes
- Thomas Oberlin, ISAE-Supaero
Chair holder :
Nicolas Dobigeon : Toulouse INP, IRIT
Senior collaborating researchers
Mathieu Fauvel, INRAE
Cédric Févotte, CNRS
Jodi Inglada, Cnes
Thomas Oberlin, ISAE-Supaero
Senior researchers:
Elsa Cazelles (CNRS, IRIT),
Henrique Goulart (Toulouse INP, IRIT),
Julien Michel (CNES),
Mickaël Savinaud (CS), Silvia Valero (UT3, CESBIO)
PhD students:
Valentine Bellet (since 2020/10),
Colin Decourt (since 2010/10),
Florentin Coeurdoux (since 2010/10),
Yo.l Zerah (since 2020/10)
Visiting researchers:
V. Tan (National University of Singapore),
N. Gillis (UMons)
- GdR ISIS & CNES 1-day meeting “Neural networks for inverse problems in satellite imagery”, 2021/09.
- CIMI-ANITI School on Optimization, 2021/09
- SMAI Mini-symposium “Optimal transport for statistical inference”, 2021/06
- Mini-workshop, “Optimization and Statistics”, 2020/09
- Data Sciences for Geosciences 2020, 2020/01
- Invited talk, Future Intelligence, 2021/06
- Talk, Mathematics and Image Analysis MIA, 2021/06
- Talk, Journée Nationale de l’Ingénieur 2021 – Intelligence Artificielle, IESF Occitanie-Méditerranée, 2021/03
- Plenary speaker, IEEE IPAS 2020, 2020/12
- Scientific advisory board & co-author, “Le Petit Illustr. Intelligence Artificielle”, co-edited by La Dépêche du Midi and CNRS, 2020/12
- Talk, IMA Workshop on Optimal control, optimal transport, and data science, 2020/10
- Invited talk, “Journée scientifique #DigitAg Intelligence artificielle en agriculture”, 2020/02
- [Ferraris2019] V. Ferraris, N. Dobigeon, Y. Cruz Cavalcanti, Th. Oberlin and M. Chabert, “Coupled dictionary learning for unsupervised change detection between multimodal remote sensing images,” Computer Vision and Image Understanding, vol. 189, no. 102817, Dec. 2019
- [Ferraris2020] V. Ferraris, N. Dobigeon and M. Chabert, “Robust fusion algorithms for unsupervised change detection between multi-band optical images – A comprehensive case study,” Information Fusion, vol. 64, pp. 293-317, Dec. 2020.
- [Guilloteau2020a] C. Guilloteau, Th. Oberlin, O. Berné and N. Dobigeon, “Hyperspectral and multispectral image fusion under spectrally varying spatial blurs – Application to high dimensional infrared astronomical imaging,” IEEE Trans. Computational Imaging, vol. 6, pp. 1362-1374, Sept. 2020.
- [Guilloteau2020b] C. Guilloteau, Th. Oberlin, O. Berné, É. Habart and N. Dobigeon, “Simulated JWST datasets for multispectral and hyperspectral image fusion,” The Astronomical Journal, vol. 160, no. 1, June 2020.
- [Oberlin2021] T. Oberlin and M. Verm, “Regularization via deep generative models: an analysis point “, arXiv, 2021.
Know more
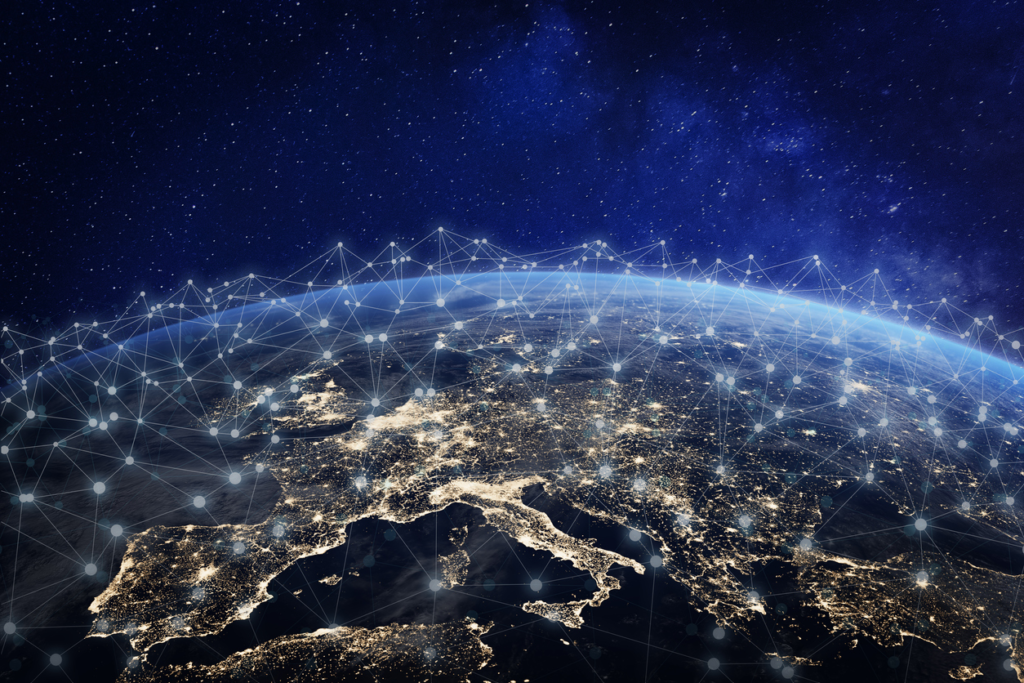
AI, is it all about data?
An Exploreur article about Nicolas Dobigeon's work