Efficient algorithms and Data Assimilation for Computationally Efficient Constrained Advanced Learning
Objectives of the chair
The objective of the chair is to promote a synergy between data assimilation and machine learning to study new algorithms as well as their efficient implementation on modern computer architectures. With regard to AI, our goal is to study methods of introducing physical constraints into machine learning algorithms, and to prove both theoretically and practically their performance.
Program Acceptable AI
Themes: Safe design and embeddability | Data & anomaly | AI & physical models | Optimization and game theory for AI
Chair holder: Serge Gratton (Toulouse INP – IRIT)
Collaborators
Pierre Boudier (ANITI)
Alfredo Buttari (CNRS)
Selime Gürol (Cerfacs)
C. Lapeyre (Cerfacs)
Data Assimilation (DA) is an ubiquitous tool for making predictions in complex systems, whether they are large-scale systems such as those derived from earth observation or systems with few degrees of freedom, such as autonomous ground or space vehicles. Performance in prediction accuracy must be obtained by using as sparingly as possible the available computing resources, whether they are hosted in computing centers or embedded in autonomous systems.
It is important to note the central difference with Machine Learning (ML): we are talking about spatial and temporal prediction that take advantage of the knowledge of time dependent physical models. The question could be rephrased in this way: how can ML improve the performance obtained by the well-established technique of DA, both in accuracy and in the use of computational resources (High Performance Computing)
Data assimilation involves making predictions from evolutionary equations that are fitted using observational data. This discipline has grown enormously in recent times, notably relying on the use of statistical sampling techniques.
This emergence has been stimulated by strong societal needs in advanced applications such as meteorology and geosciences in general, but also other sectors such as neutronics, mechanics to name a few. It is also observed that the mathematical complexity of modern evolution models increases such that monitoring systems in real time is a challenge in many applications. In this regard, the important questions to be resolved relate to a good management of the non-linearity of the models, to the attenuation of the linear and Gaussian assumptions which are at the heart of the most recent algorithms, and to the efficient use of calculation resources.
Backed by a very general statistical theory, deep learning algorithms make it possible to make predictions on a wide variety of data sets and achieve impressive performance, both in terms of precision and execution time. In many applications such as image and speech processing, for example. The key is the expressiveness of deep neural networks, which makes it possible to address very nonlinear functions, the use of rich databases, and the implementation of algorithms on computational architectures dedicated to these prediction tasks.
Chair holder : Serge Gratton, INP-IRIT
Co-chairs :
- Alfredo Buttari (CNRS, IRIT)
- Corentin Lapeyre (Cerfacs)
Senior researchers :
- Pierre Boudier (Nvidia)
- Selime Gurol (Cerfacs)
PhD students
- Th. Beuzeville (Cifre Atos)
- S. Jerad (ANITI)
- V. Mercier (Cifre BRLi)
- M. Peyron (Cifre Atos)
Post-docs
- A. Fillion (oct. 2019, oct.2021)
Visiting researchers:
- Ph. Toint (University of Namur, Belgium)
- COAP 2019 Best Paper award: Paper of S. Gratton, C. W. Royer, L. N. Vicente, and Z. Zhang. Comput. Optim. Appl. 77, 617–621 (2020).
- DAN–An optimal Data Assimilation framework based on machine learning Recurrent Networks P Boudier, A Fillion, S Gratton, S Gürol. Submitted to SIAM
- Latent Space Data Assimilation by using Deep Learning. Mathis Peyron, Anthony Fillion, Selime Gurol, Victor Marchais, Serge Gratton, Pierre Boudier, and Gael Goret, under revision for QJRMS.
- A coarse space acceleration of deep-DDM, Valentin Mercier, Serge Gratton, Pierre Boudier. Submitted to Neurips 2021
- An algorithm for the minimization of nonsmooth nonconvex functions using inexact evaluations and its worst-case complexity. S Gratton, E Simon, Ph.L.Toint, Mathematical Programming, 1A, 2-24, 2020
- Adversarial attacks via backward error analysis. Th.o_Beuzeville, Pierre Boudier, Alfredo Buttari, Serge Gratton, Theo Mary, St.phane Pralet. Submitted to Neurips 2021
Know more
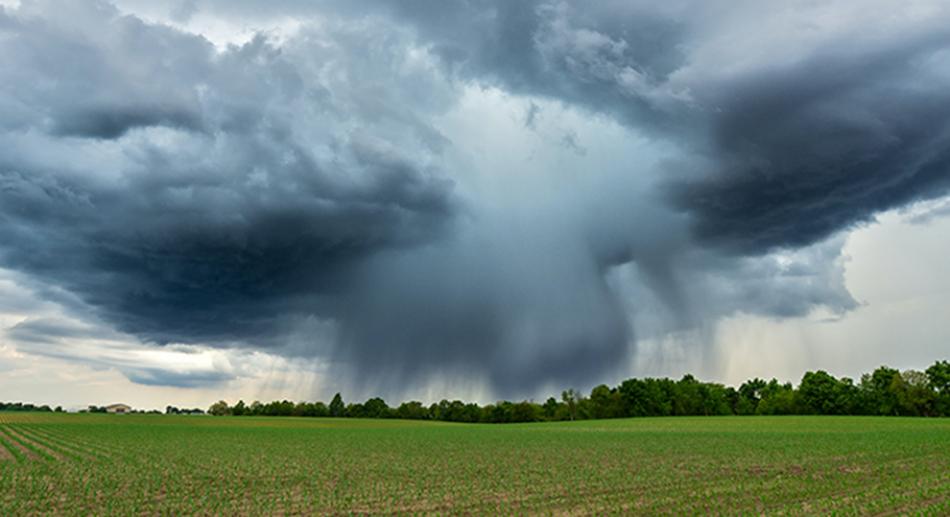
Artificial intelligence to boost forecasts of all kinds.
What is the relationship between data assimilation, weather forecasting and artificial intelligence?
What are the links between oceanography, a prediction confidence index and machine learning?